Integrating AI and Machine Learning into SIEM Systems
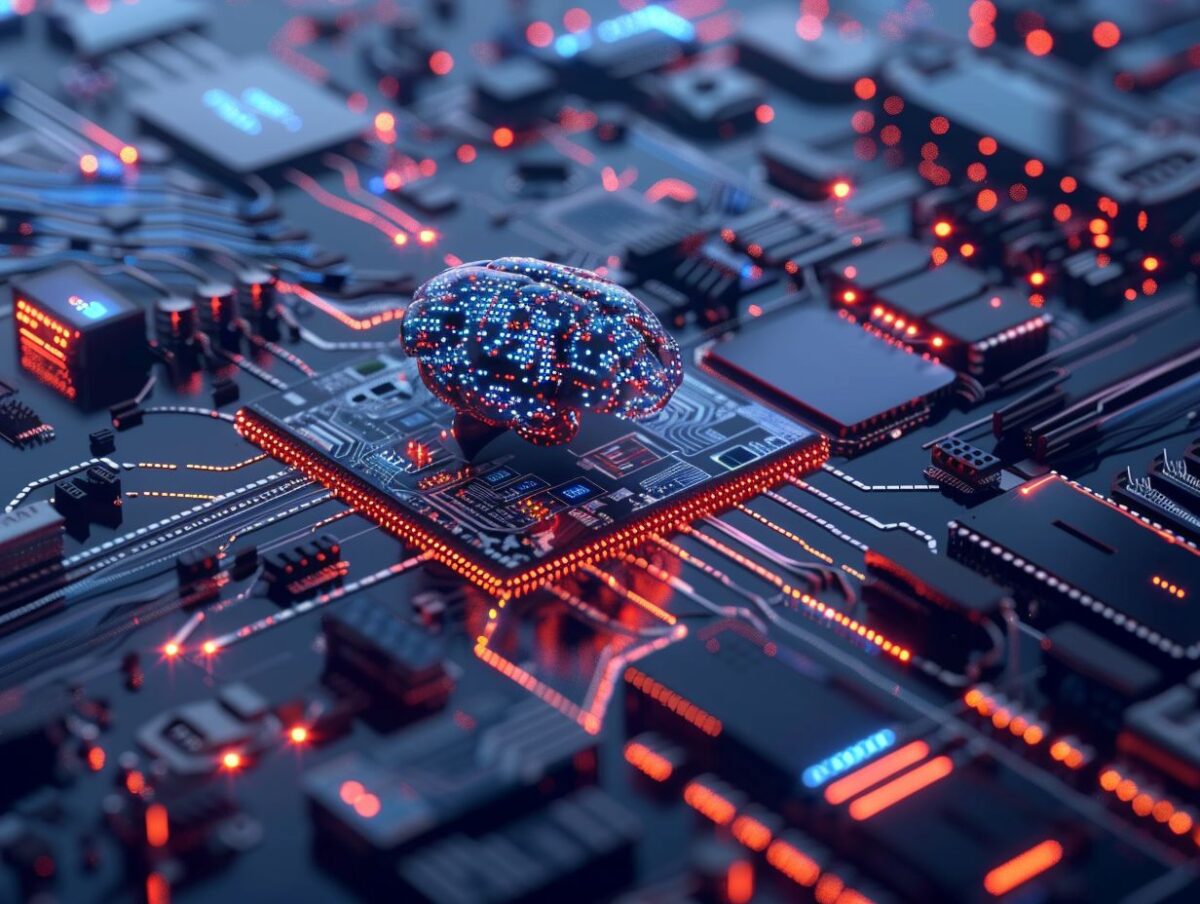
In today’s fast-paced digital world, cybersecurity threats are constantly evolving, making it crucial for organizations like yours to stay ahead of potential risks.
Enhancing security measures can be achieved by integrating Artificial Intelligence (AI) and Machine Learning into Security Information and Event Management (SIEM) systems.
This article will explore the role of AI and Machine Learning in SIEM, the benefits and challenges of this integration, best practices for implementation, real-world examples, and predictions for the future of SIEM with AI capabilities.
Join us as we delve into the latest advancements in cybersecurity technology tailored to your organization’s needs.
Key Takeaways:
Understanding SIEM Systems
Understanding SIEM (Security Information and Event Management) Systems is crucial for organizations seeking to enhance their cybersecurity posture. SIEM systems play a critical role in monitoring and analyzing security events within an organization’s network infrastructure.
These systems facilitate real-time monitoring of network activities, log collection, and alert generation based on predefined rules. Through data correlation from multiple sources, SIEM can detect potential security incidents, offering a comprehensive overview of the organization’s security landscape.
In terms of incident response, SIEM enables security teams to promptly identify and address threats, thereby reducing the impact of breaches. Leveraging advanced technologies like machine learning and automation further enriches SIEM capabilities, enabling more precise threat detection and swifter response times.
What is SIEM and How Does it Work?
SIEM (Security Information and Event Management) is a comprehensive approach to security management that combines the capabilities of security information management (SIM) and security event management (SEM). It works by collecting and correlating data from various sources to identify potential security threats.
By analyzing log and event data in real-time, SIEM systems provide security teams with valuable insights into network activity and potential vulnerabilities. Leveraging AI and machine learning algorithms, these systems are able to detect patterns and anomalies that may indicate a security breach. SIEM solutions offer automated response capabilities, allowing for immediate action to be taken when a threat is detected.
The integration of threat intelligence feeds further enhances the system’s ability to stay ahead of emerging threats and evolving attack techniques, making SIEM a crucial component in modern cybersecurity strategies.
The Role of AI and Machine Learning in SIEM
The integration of Artificial Intelligence (AI) and Machine Learning (ML) technologies in SIEM systems has revolutionized cybersecurity practices. AI and ML enable SIEM solutions to analyze vast amounts of data, identify patterns, and automate response actions.
These advanced technologies play a crucial role in enhancing threat detection by continuously learning and adapting to new cybersecurity challenges. With the use of sophisticated algorithms, SIEM platforms powered by AI and ML can normalize and enrich data, ensuring accurate and efficient analysis of potential security incidents. This capability enables organizations to proactively detect anomalies, monitor network activities, and respond to threats in real-time, thereby significantly improving their overall security posture.
Benefits of Integrating AI and Machine Learning
The integration of AI and Machine Learning in SIEM systems offers a range of benefits to organizations, including enhanced threat detection capabilities, reduced response times, and improved efficiency in handling security incidents.
By leveraging AI and ML, you can experience advanced anomaly detection, enabling the identification of abnormal behaviors and potential security threats in real-time. These technologies can also aid in the automation of routine security tasks, allowing security teams to focus on more strategic initiatives.
Through continuous learning and adaptation, AI and ML algorithms can evolve to better identify evolving threats and patterns, enhancing the overall security posture of your organization. The predictive analysis provided by AI algorithms can help in preemptively addressing potential security breaches before they escalate.
Challenges and Limitations
Despite the advanced capabilities they offer, the integration of AI and Machine Learning in SIEM systems also presents challenges and limitations that organizations need to address. These may include concerns about data privacy, model accuracy, and the need for continuous monitoring and updates.
Organizations must also navigate the intricacies of ensuring the scalability of AI and ML models within their existing SIEM infrastructure. Managing the sheer volume of data that these systems analyze, while ensuring real-time responses to security incidents, can strain resources and affect performance. It is essential for organizations to strike a balance between leveraging the power of AI and ML for threat detection and mitigation, while maintaining the reliability and speed of their SIEM solutions. Overcoming these challenges requires careful planning, resource allocation, and a thorough understanding of both the technology and the cybersecurity landscape.
Implementing AI and Machine Learning in SIEM
The implementation of Artificial Intelligence (AI) and Machine Learning (ML) in SIEM solutions requires a strategic approach that incorporates advanced algorithms, robust techniques, and scalable components to ensure effective threat detection and response mechanisms.
By integrating these cutting-edge technologies, you can enhance your security posture by analyzing vast amounts of data in real-time, identifying anomalies and potential threats that traditional systems might overlook. The significance of technology integration lies in the ability to leverage AI and ML to automate processes, providing quicker responses to evolving cyber threats.
Network analysis plays a pivotal role in identifying patterns and behaviors, allowing for proactive threat mitigation strategies. Incorporating cybersecurity algorithms and techniques ensures that SIEM systems can adapt and learn iteratively, improving accuracy and reducing false positives.
Best Practices and Strategies
For the optimization of AI and Machine Learning integration in SIEM solutions, your organization should consider adopting best practices and strategies that emphasize continuous improvements, efficient use of techniques, and the application of advanced algorithms for proactive threat detection and response.
Utilizing the capabilities of AI and ML enables organizations to establish stronger security frameworks that can adapt to evolving threats in real-time. Incorporating anomaly detection algorithms supports the early identification of suspicious activities and potential breaches. Employing entity behavior analysis offers deeper insights into user actions and network behaviors, facilitating the implementation of more precise threat mitigation strategies.
Training AI and ML models with high-quality data allows organizations to enhance the accuracy of threat detection and minimize false positives, ultimately strengthening their overall cybersecurity stance.
Real-World Examples of AI and Machine Learning in SIEM
Real-world examples can showcase the practical applications of Artificial Intelligence (AI) and Machine Learning (ML) in SIEM systems, illustrating how these technologies can facilitate advanced threat intelligence, rapid incident response, and pattern recognition for automated security operations.
When considering AI and ML, cybersecurity teams benefit from the ability to analyze vast volumes of data to identify patterns and potential risks effectively. For example, a recent case study highlights how a financial institution utilized AI-driven SIEM solutions to detect anomalous behavior indicative of a ransomware attack.
Through autonomous correlation of suspicious patterns across multiple systems, the system promptly initiated automated alerts. This enabled the security team to swiftly isolate and neutralize the threat before any data loss occurred.
Case Studies and Success Stories
The utilization of AI and Machine Learning in SIEM solutions has proven to enhance efficiency, decrease false positives, and provide actionable insights in the realm of advanced cybersecurity technologies. By examining case studies and success stories from organizations that have implemented these technologies, valuable insights can be gained into the benefits they offer.
Real-world examples illustrate how cybersecurity organizations have effectively utilized AI and ML to optimize threat detection and response workflows, resulting in notable enhancements in operational efficiency. For example, a financial services firm incorporated AI algorithms into their SIEM system and experienced a 30% reduction in false positives, along with a 50% decrease in incident response time. Similarly, a healthcare provider noted a significant uptick in identifying advanced persistent threats following the implementation of machine learning models in their security operations. These success stories highlight the concrete advantages of adopting innovative technologies within the cybersecurity domain.
Future of SIEM with AI and Machine Learning
The future of Security Information and Event Management (SIEM) systems with Artificial Intelligence (AI) and Machine Learning (ML) holds immense potential for predictive analytics, deep learning capabilities, Natural Language Processing (NLP) advancements, and User and Entity Behavior Analytics (UEBA) innovations.
These advancements in SIEM systems leveraging AI and ML technologies are expected to revolutionize cybersecurity practices by enabling organizations to proactively detect and respond to emerging threats. With the integration of predictive analytics, organizations can anticipate potential security incidents before they occur, thereby bolstering their defense mechanisms. Deep learning algorithms will enhance the system’s ability to identify complex patterns and anomalies, while NLP enhancements will facilitate better communication and understanding of security alerts. UEBA innovations will provide more granular visibility into user behavior, helping organizations to swiftly identify malicious activities and potential insider threats.
Predictions and Potential Developments
Predictions and potential developments in the realm of AI and Machine Learning within SIEM systems indicate a shift towards more advanced technology integration, enhanced network analysis capabilities, automated response mechanisms, and proactive cybersecurity measures.
These advancements are set to revolutionize the cybersecurity landscape by enabling organizations to detect and respond to threats in real time, ensuring faster and more accurate threat identification. Improved AI algorithms will enhance the accuracy of anomaly detection, while ML integration will facilitate predictive analytics for preemptive threat mitigation. With the automation of incident response powered by AI, security teams can focus on strategic decision-making and threat intelligence, ultimately strengthening overall cybersecurity defenses.
Frequently Asked Questions
What is the benefit of integrating AI and Machine Learning into SIEM systems?
Integrating AI and Machine Learning into SIEM systems can greatly enhance the detection and response capabilities of the system. AI and Machine Learning algorithms can analyze large amounts of data in real-time, identifying patterns and anomalies that human analysts may miss. This can improve the overall security posture of an organization.
How do AI and Machine Learning algorithms work in SIEM systems?
AI and Machine Learning algorithms use advanced techniques such as natural language processing, deep learning, and neural networks to analyze data and learn from it. They can identify patterns and anomalies in data, classify events, and make decisions based on historical data and user-defined rules.
What are the challenges of integrating AI and Machine Learning into SIEM systems?
One of the main challenges is the need for high-quality data. AI and Machine Learning algorithms require large amounts of data to train and make accurate decisions. This means organizations must have robust data collection and storage processes in place. Additionally, the algorithms need to be constantly monitored and tuned to ensure they are providing accurate results.
How can integrating AI and Machine Learning into SIEM systems improve threat detection?
With AI and Machine Learning, SIEM systems can continuously learn from past events and adapt to new threats and attack techniques. This can improve the accuracy and speed of threat detection, allowing organizations to respond to incidents more quickly and effectively.
Can AI and Machine Learning replace human analysts in SIEM systems?
No, AI and Machine Learning algorithms cannot completely replace human analysts. While they can automate certain tasks and improve efficiency, human analysts are still needed to make critical decisions and provide context to the data. A combination of AI and Machine Learning with human expertise can provide the best results in SIEM systems.
What are some potential risks of integrating AI and Machine Learning into SIEM systems?
One potential risk is the possibility of false positives or false negatives. AI and Machine Learning algorithms may make inaccurate decisions if not properly trained or monitored. There is also the risk of bias in the algorithms, which can lead to discriminatory results. Organizations must carefully evaluate and test the algorithms before integrating them into their SIEM systems.